Data, Innovation and Opportunity
Investments in modernization may unlock business value in unexpected places September 2020In the Spring 2020 issue of The Actuary, data was the theme running through many of the articles. In his editorial, Martin Snow, FSA, MAAA, spoke of the new frontier of artificial intelligence (AI) and machine learning (ML), and the challenges of overcoming the inertia with existing processes occupying valuable time and resources. Over the years, the actuarial profession has adapted well to embrace technology and thrive. Our current environment is no different, and new techniques should not be viewed as a risk but as an opportunity. However, the question is: How do we move on this opportunity?
Outside of the actuarial domain, data is a common theme, too. The Economist published an article discussing the data economy with the bold title, “The World’s Most Valuable Resource Is No Longer Oil, but Data.”1 Unlike oil, data cannot be burned to produce momentum. It requires a considerable amount of science to go from raw material to concrete value. A Gartner article predicted that through 2020, 80 percent of AI projects “will remain alchemy, run by wizards whose talents will not scale in the organization.”2 This points to an opportunity for the actuarial profession to step in. Actuaries have established a rigorous scientific approach to their work, one that is proven to scale and is leveraged by the insurance industry.
Using data for AI and ML itself is not a strategy, though. The disruption created by companies like Amazon, Uber, Microsoft, Google, Facebook and Tesla was not brought about by setting out to do data science. Google’s mission is “to organize the world’s information and make it universally accessible and useful.” Tesla’s is “to accelerate the world’s transition to sustainable energy.” And Microsoft strives “to empower every person and every organization on the planet to achieve more.” These companies are experts at creating value from data; but, more important, they have a clear purpose and know how to use data to deliver demonstrable impact.
The predicted failure rate of big data projects is sobering: “Through 2022, only 20 percent of analytic insights will deliver business outcomes,” according to Gartner.3 Bill Gates is said to think that most people overestimate what they can achieve in the short term and underestimate what they can do in the long term. In data projects, the situation is almost reversed; there’s a short-term focus on gathering data to service a future need that is only vaguely conceptualized. What engineering practices have taught us is that you must iterate toward a goal. Getting to the moon was an audacious goal; many said it was impossible. But NASA solved the problem by following a rigorous scientific process based on experiments. They took an incremental approach to get to the moon, one launch at a time—they didn’t shoot for the moon in one go.
This preamble is to say: ML and AI are an opportunity, actuaries are well-positioned to help, and success comes from driving toward valuable business outcomes using a rigorous iterative process. What next?
Customer Focus
Lowering the cost of doing business is not exciting. Paying down the technical debt4,5 that is holding back innovation is a potential source of long-term competitive advantage, but it’s often overlooked. It is not an option to just stop doing the work we must do. It’s hard to start over, from a blank sheet of paper, especially in heavily regulated industries like insurance. In the United Kingdom, Starling Bank, a FinTech and “challenger bank” founded in 2016, is doing well starting from a blank sheet, with 1.4 million personal accounts and 155,000 business accounts.6 It is unencumbered by legacy systems, which is the basis of one of its competitive advantages: “Starling’s world-class tech reimagines banking for life today, putting the tools people need to feel good about money in the palm of their hand.”7 InsurTech has startups in health and property and casualty, but, looking at the top 15 companies in January 2020, there are none of note in the life insurance sector.8
Although regulation remains a barrier to innovation, it may not make for a level playing field. For example, the Volkswagen Group caught regulators’ attention for cheating emissions standards, and they are now going to be subject to increased scrutiny going forward, while a different automotive company might not be. Mistakes of the past are a legacy that cannot be easily undone, and new companies simply have not made big mistakes yet. Although the technology startup mindset of “move fast and break things” (the motto of Facebook’s Mark Zuckerberg) really can’t apply in insurance, the ability to take a risk needs to be there to bring about lasting change. Insurers need to lay the groundwork for regulatory approval of new products and new business practices that may emerge from the continued adoption of new technologies, and actuaries are key parts of this process.
To compete in a digital marketplace, incumbent insurers may create innovation hubs that look for ways to unlock the value of technological advances. For example, Aviva has a digital garage that “takes the IT department concept to the next level with a fast-paced agency atmosphere dedicated to producing mind-boggling digital customer experiences, quickly and often.” The focus of much of this innovation is on growing the top line. This has a material impact when the cost of customer acquisition is high, and it is also easier since it’s not deeply integrated into a complex value chain—instead, it’s at the start of one.
Lowering the cost of customer acquisition also can remove barriers to completing a sale. Amazon’s “one-click ordering” is a good example of removing steps in closing a sale, and it has set customer expectations. This is particularly relevant to us, as it’s often said millennials are impatient and they are the future buyers of insurance.
In life insurance, automated underwriting removes the need for intrusive medical tests, which take weeks. If you can move faster than your competition, you’re more likely to win the client, especially today when people expect immediate results. Speeding up claims processing by using images uploaded from mobile phones provides a much better customer experience. It reduces the time it takes to settle a claim, which also lowers cost and fraud. Devices that track customer behavior—for example, driving style or number of steps per day—can result in lower premiums for the customer, because the risks the insurer and individuals are exposed to are lower. Each of these examples is customer centric and data dependent—and infused with AI/ML.
Nudge theory, from behavioral economics, proposes that positive reinforcement and indirect suggestions may influence the behavior and decision-making of groups of individuals. Thinking of customer behavior as something that can be modeled and influenced is a powerful concept, which creates an opportunity to use predictive modeling to identify and better serve profitable customers. This should enhance long-term profitability through the improved marketing and retention that results from better placement of distribution centers and alignment of agent incentives.
These approaches provide concrete value quickly and have solid short-term goals where success is easily measured. However, they do not bring about organizationwide transformation.
The advantage a startup has over an incumbent is that it is a digital native—technological innovation is infused throughout the organization and not limited to pockets of excellence, partners or InsurTech acquisitions. Tesla does not have a data center of excellence; it is one. Tesla was founded in 2003, and in 2020 became the world’s most valuable car company with a $208 billion valuation.9 The company has taken an engineering first view to all areas of its business, from building better batteries (and open sourcing the specifications), to supply chain, automated driving assistance and vehicle safety. Tesla is entering the automotive insurance market to offer lower premiums than traditional insurers.10 Tesla can offer these lower premiums thanks to the huge amounts of driver data it collects and analyzes. Additionally, Tesla benefits from breaking down traditional silos between businesses. It can optimize repair costs to reduce the liability and embed driver safety aids to minimize the risk of an accident. This kind of disruption breaks traditional business models and creates a differentiated advantage that cannot be easily replicated. There is a great deal of benefit to the consumer, as the customer experience is streamlined, both in sales and in claims. And, more important, the aligned incentives mean car journeys are safer.
In times of economic turmoil, as we’re experiencing today with COVID-19 and might experience in the following recession, there will be a knock-on impact. Fewer customers with surplus income could lead to a decline in new business and increased lapses. Are there other areas of focus that could help bring about a broader digital transformation? Could those benefits be more impactful during a downturn?
Back-end Focus
With back-office transformation, it is possible to go beyond the focus on customer experience. Since expenses incurred are significant in the back office, driving down the cost of policy administration is a valuable goal. Robotic process automation (RPA) reduces the time it takes to execute complex processes and improves quality and agility from early insight, which is especially valuable in times of market turmoil. The actuarial engine room is a good place to innovate if you want to unlock intellectual capital, drive out technical debt and reduce expenses. Regulatory burden is increasing, as are the complexity of products, and it’s not an insignificant challenge or investment to reduce the total cost of ownership of an insurance policy. It is also likely that the burden of regulatory change will be felt more severely due to technical debt and accidental complexity.11,12 Having systems that are hard to adapt inhibits agility. This increases the cost of change or likelihood of missed opportunities, especially in an environment of economic or regulatory change, and is yet another example of how a startup could have a significant advantage over an incumbent.
Looking at a life insurer’s modeling function reveals some interesting parallels to the world of the modern startup’s data strategy. Leaning into that to organically build muscle for data science, ML and AI bring another business function into the new frontier. Marrying the digital culture of the frontend of the business with the back office could lead to a revolutionary change after which everyone thinks as a digital native and speaks the same language—and that synergy may provide unexpected business outcomes.
Actuarial Modeling and Data Science
How much does the actuarial modeling team have in common with a modern data science team? What can they learn from each other, and how could commonality create synergy? How might that be exploited?
Software engineering has had a concept of DevOps (combining software development and IT operations to shorten systems development life cycle and increase quality) for several years. MLOps, ModelOps or modern data science is similar and brings data scientists and operations professionals together to manage the production ML life cycle. Figure 1 is an example of the process from Microsoft.
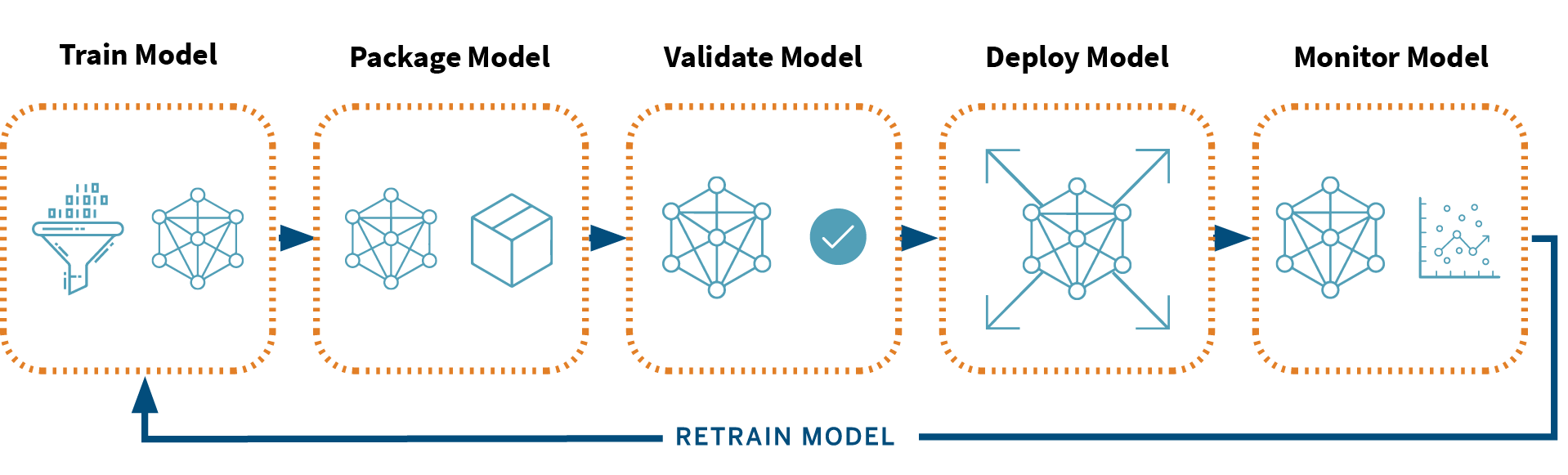
Source: MLOps with Azure ML. Microsoft (accessed June 23, 2020).
This process is data centric and sits within the context of a data platform that delivers curated input to models. SAS defines a “centralized repository” where “collaborative, cross-functional teams capture and store model information and related metadata in a single location.”13 The actuarial modeling process is similarly data centric, and data is often cited as a major cause of concern for the actuarial modeling teams.
Taking a step back from the technology view and thinking about the theoretical process, you see a scientific process akin to what many actuaries do today: Domain experts run a series of experiments to validate a theoretical model built using ML and pre-existing data.14
Actuarial processes can benefit a great deal from the contemporary concepts of DevOps and ModelOps, with only minimal change. A modern end-to-end architecture, similar to what is illustrated in Figure 2, automates complex processes and also establishes rigor in the development life cycle. This reduces cost of ownership, increases accuracy and removes manual controls that are costly to demonstrate during an audit.
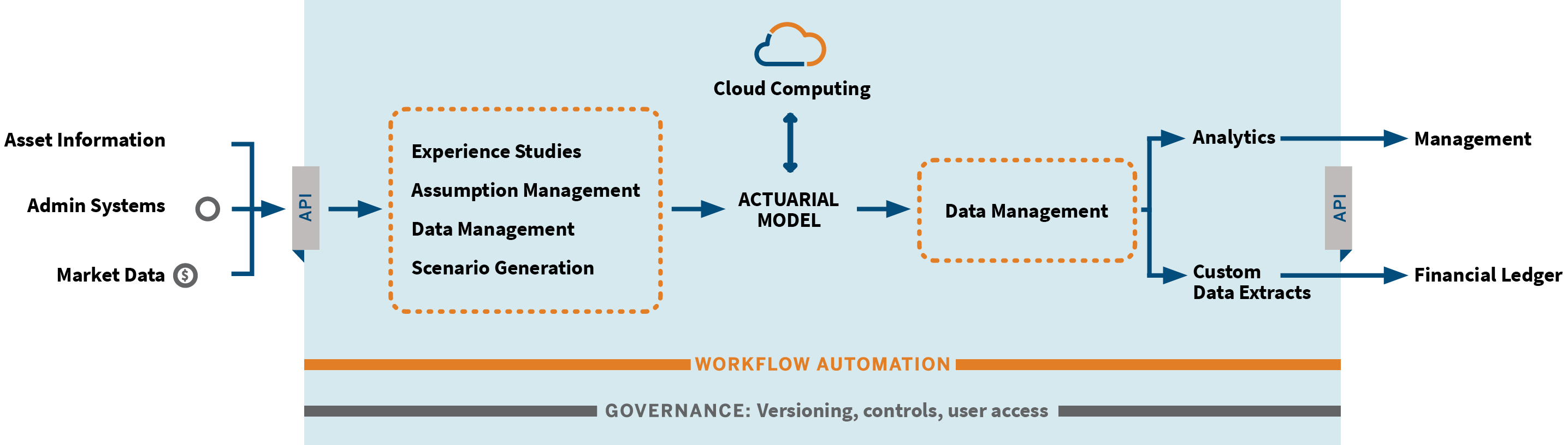
Source: Milliman
An opportunity associated with transforming toward engineered processes, common in software engineering and productized ML models, is synergy in investments across the business. One such example is observed from the widespread adoption of agile methods beyond the software engineering teams. It is now quite common to find Scrum-based agile processes in several areas of change in an organization. The foundational view of a process like Scrum is one of scientific rigor—an empirical process model. This model provides control through frequent inspection and adaption of processes that are imperfectly defined and initially generate unpredictable or unrepeatable results. Said differently, the model asserts that knowledge comes from experience.15 Agile methods, like Scrum, have provided a framework for companies to deliver incrementally and realize a return on investment (ROI) sooner. It’s like NASA getting to the moon: one experiment at a time, using data to take a better step forward.
The standardization of tools across an entire organization from the top down is expensive and onerous to roll out. It’s also counterproductive, as everyone has different requirements. Indeed, the actuarial modeling core is very specialized. Thought leaders in modern data warehousing, such as Netflix and Uber, are choosing to leverage open-source technologies and not the existing enterprise solutions. These open-source solutions are available from each of the major cloud providers in the form of a platform as a service. Google gave away huge amounts of value in the form of open-sourced frameworks, like TensorFlow, and pretrained models for common tasks like image and voice recognition. Companies can connect and combine them with their own intellectual property to deliver their unique services to the market very quickly. It’s also interesting to observe the partnerships of cloud providers, such as the partnership between Microsoft and SAS,16 which will bring well-established tools into this open-source cloud era.
As each cloud vendor provides a broad range of technologies to innovate with on a single connected platform, there are very few barriers to getting started. The emerging cloud first strategy of many insurers should mean that technology decisions are democratized, and business units can more easily invest in tools connected to their cloud data lake. As those new use cases contribute more data back to the lake, there is organic growth and increased opportunity to leverage the data across organizational silos.
Bringing It All Together
There is indeed a new frontier. More companies are undertaking scientifically rigorous data processes, which is in the wheelhouse of actuarial teams. There are a lot of benefits to increasing the top line, but there are just as many in improving the bottom line. The challenging times we’re in provide an opportunity to optimize the back office by leveraging the very same approaches that drive impact to the top line. The long-term value is a unified, digitally transformed organization that is less encumbered by technical debt and more agile, multidisciplinary and collaborative.
There is an additional competitive advantage to be gained from building sophisticated models for policyholder behavior or for other actuarial assumptions. This advantage might be leveraged by reinsurers or potential merger and acquisition (M&A) deals as companies look to identify attractive blocks of business for acquisition or to divest unattractive blocks. These same models may be applicable to attracting and retaining customers at the front end. Before you know it, you may have marketing leaning on lapse studies conducted by the actuarial team, or customer retention teams leveraging the actuarial model to assess customer value when making decisions about reducing premiums to retain profitable customers. In short, democratization of both data and models could have a far-reaching organizational benefit.
It is critical to focus on delivering business outcomes and not fixate on the technology or the promise of data. This is also a perfect opportunity to build a “digital native” culture to facilitate transformation across the organization, bringing in actuarial talent instead of keeping it in a silo. Peter Drucker said that “culture eats strategy for breakfast,” and McKinsey & Company research found that “digital transformations require cultural and behavioral changes.”17 The McKinsey & Company research suggests that the key factors for successful digital transformation are leadership, building for the future, empowering people to work differently using collaborative methods and having the right tools. This is a lot for any company to manage, but those companies that address all of these factors in a coherent way are the companies that will shape the future of insurance.
References:
- 1. The World’s Most Valuable Resource Is No Longer Oil, but Data. The Economist, May 6, 2018 (accessed June 23, 2020). ↩
- 2. White, Andrew. Our Top Data and Analytics Predicts for 2019. Gartner, January 3, 2019 (accessed June 23, 2020). ↩
- 3. Ibid. ↩
- 4. Technical debt is a metaphor coined by Ward Cunningham to describe the cost of additional work due to the accumulation of suboptimal solutions to problems that slow future progress, akin to the accumulation of interest on a loan. ↩
- 5. Fowler, Martin. TechnicalDebt. MartinFowler.com, May 21, 2019 (accessed June 28, 2020). ↩
- 6. O’Hear, Steve. Existing Backers Put Another £40M Into U.K. Challenger Bank Starling. TechCrunch, May 28, 2020 (accessed June 23, 2020). ↩
- 7. About Starling Bank. Starling Bank (accessed June 23, 2020). ↩
- 8. Grones, Geraldine. Top 15 InsurTech Companies in the U.S. Insurance Business America, January 17, 2020 (accessed June 6, 2020). ↩
- 9. Klebnikov, Sergei. Tesla Is Now the World’s Most Valuable Car Company With a $208 Billion Valuation. Forbes, July 1, 2020 (accessed July 30, 2020). ↩
- 10. Sonnemaker, Tyler, and Rapier Graham. Elon Musk Says Tesla Is Creating a “Major Insurance Company” After Its Botched Rollout in California Last Year. Business Insider, July 23, 2020 (accessed July 30, 2020). ↩
- 11. Accidental complexity is a term coined by Fred Brooks in his 1975 paper “No Silver Bullet,” which describes complexity introduced by the engineers building a system—which is not inherent to the problem domain. ↩
- 12. Brooks, Frederick P. Jr. 1995. The Mythical Man-Month: Essays on Software Engineering. Addison-Wesley. ↩
- 13. Operationalizing Analytics: What Is ModelOps? SAS Institute Inc. (accessed June 23, 2020). ↩
- 14. IT Central Station. Modern Data Science: Best Practices for Predictive Analytics, IBM, 2018 (accessed June 23, 2020). ↩
- 15. Schwaber Ken, and Mike Beedle. 2002. Agile Software Development with Scrum. Prentice Hall. ↩
- 16. Microsoft News Center. SAS and Microsoft Partner to Further Shape the Future of Analytics and AI. Microsoft, June 15, 2020 (accessed June 23, 2020). ↩
- 17. Unlocking Success in Digital Transformations. McKinsey & Company, October 29, 2018 (accessed June 26, 2020). ↩
Copyright © 2020 by the Society of Actuaries, Chicago, Illinois.