Fighting Inertia
Is the pension risk transfer market the next foray for predictive analytics? February/March 2017The field of predictive modeling has been around for quite some time. It has been a mainstay in the property and casualty (P&C) insurance world for decades, particularly in what might be called the out-of-the-money risk space. For certain lines like automobile insurance, voluminous reams of credible data on accident claims have typically existed, and this data was generally sufficient for pricing and reserving actuaries to gain comfort with the claims frequency and severity assumptions they used.
Such credible data did not exist, however, for coastal homeowners insurance where the frequency and severity of claims could vary wildly as a result of unpredictable natural disaster activity, for example. Unlike auto insurance actuaries (and, similarly, life actuaries who typically have also had reams of credible data on mortality), “tail risk” actuaries have not relied as much on past data (“the then”) in pricing policies and setting reserves. They instead generally had to rely more on the predictive power of observable variables (“the now”) related to the exposure insured, which has required the use of predictive modeling.
In its simplest form, predictive modeling is an exercise of collecting lots of data about the characteristics of an insured exposure and running multivariable regressions to determine which variables, in which combinations, have predictive power. It’s a pretty straightforward exercise—for the average actuary, at least.
As time progressed and modeling capabilities improved across the insurance industry, predictive modeling started to be used in the health insurance space by the turn of the century. Health actuaries began to realize that, for example, collecting data on someone’s medical records, health history, exercise habits, shopping habits and risk appetite (e.g., tendency to gamble, frequency of speeding tickets) could inform models that have significant predictive power. The next logical step for health actuaries was to incorporate this modeling into health insurance pricing and reserving.
Over the last decade and a half, the availability of information, both public and confidential, has increased exponentially, which has caused the predictive power of these models to increase in turn. It was only a matter of time, and indeed a foregone conclusion, that these models would begin to be utilized in the life insurance space to help inform mortality and longevity assumptions. True to form, we are seeing many applications of predictive modeling inform underwriting, pricing and marketing decisions in the life insurance space more and more. What does the future hold? What is the next great foray for predictive analytics? Perhaps it is the “land of vanishing inertia”—the U.S. pension risk transfer (PRT) market.
The PRT Market—Past, Present and Future
In my view, pension risk across the globe has been largely mismanaged macroeconomically for many decades, due in large part, as noted previously, to some forces of inertia fighting against the transfer of risk into the hands of the most appropriate managers of that risk. That risk is largely financial and actuarial in nature. Modern insurers have been researching, modeling and managing actuarial and financial risks for decades, whereas, to plan sponsors—particularly those that are nonfinancial companies—pension risk is typically an unwanted distraction to an organization’s business plan and often a source of seemingly uncontrollable uncertainty. The mission, vision and values of these organizations simply don’t tend to incorporate pension risk management. Nor should they. These companies do things like make the tiles that end up on your kitchen counter; they make sure you have clean running water 24/7; they make sure we fly the friendly skies in a safe and efficient fashion. Pension risk doesn’t belong in their wheelhouse.
Now, let’s add a few additional points to the narrative. The cost to administer pension plans is becoming increasingly burdensome at many plan sponsor organizations. Investment management fees for plan sponsors are often significant and vaguely disclosed. Many life insurers are finding it increasingly challenging to drive top line revenues and achieve target returns on capital with traditional products. When compared to the menu of modern life insurance product liabilities that insurers hold, PRT liabilities are squeaky clean (for example, the exposure to policyholder behavior is either minimal in comparison or nonexistent). Taking all of that into consideration, it is no surprise that the PRT market continues to exhibit significant growth and is poised to do so for many years to come.
PRT markets around the globe vary naturally in size, scope and nature due primarily to different regulatory paradigms, different cultures and risk takers with different styles and appetites.
In the United Kingdom, for example, the PRT market is relatively mature and uses several instruments (annuity buy-ins, group annuity buy-outs and longevity swaps) to help plan sponsors cover the various pension risks. Contributing to that might be the fact that the regulatory construct requires actuaries to be on both sides of the pension balance sheet (plan scheme actuaries on the liability side and trustee actuaries on the asset side).
In Canada, the PRT market is nascent, but clearly growing, with the first longevity swap transaction closing in 2015. 1
In Japan, there has been a small wave of transactions for Japanese companies that have U.S. subsidiaries with U.S. plans. These organizations might be likely candidates for PRT if and when the funded status of Japanese plans eventually improves, which would be helped by an increase in interest rates. They also have the hurdle, in certain circumstances, of needing to get buy-in from two-thirds of the covered workforce.
Irrespective of the specific country of domicile, the general themes that surround the transactions are common:
- Care needs to be taken (by regulatory bodies as well as paternalistic plan sponsors) to ensure that the insurer that assumes the pension benefit obligations is financially strong and well positioned to make good on the pension promises in perpetuity.
- From the perspective of both the plan sponsor and the insurer, the economics of the transaction must fit within their general business models.
We now turn to the U.S. market specifically to illustrate how the dynamics of the transactions work and where predictive analytics might fit.
The PRT Market in the United States
By the numbers, 2 as shown in Figure 1, the growth and growth potential is quite eye opening. We count just fewer than 20 major players that are either actively transacting in the U.S. group annuity pension buy-out market and/or demonstrating genuine appetite to transact in 2017. Those companies were responsible for the transfer of about $15 billion in pension assets and liabilities in 2016, which is no small number. Nonetheless, it is well below 1 percent of the total potential market of transferrable U.S. corporate defined benefit (DB) plan assets. In short, to the extent pricing remains attractive and capacity on the insurance side remains adequate, this market likely will be a source of safe haven growth for years to come.
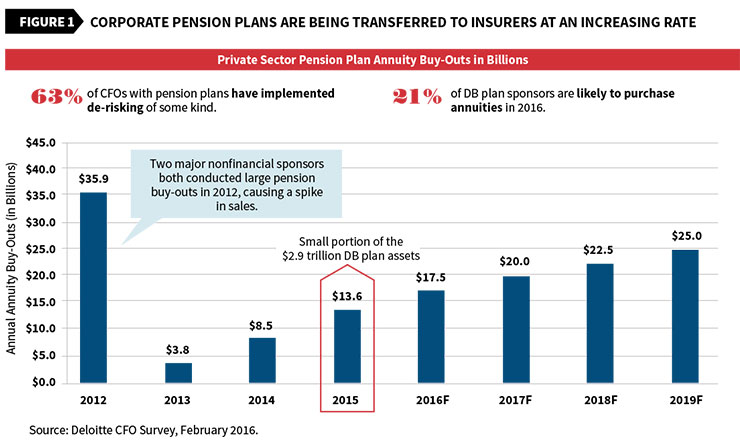
Another wrinkle to add, which is likely to continue to contribute to PRT market growth, is that the premiums that plan sponsors must pay to the Pension Benefit Guaranty Corp. (PBGC) per participant have increased exponentially and are scheduled to increase by an additional 25 percent by 2019. 3 All in all, the trends seem to point toward a bright future for this burgeoning market.
That market, at the moment, appears to be somewhat segmented, with some price competition on the lower end, some capacity constraints and a smaller field of potential writers on the larger end, and a mixed bag of structures in the middle market. The larger end, or jumbo market, transactions typically include separate account structures that transact using assets in kind (as opposed to cash). In these situations, insurers take a look at the assets held by plan sponsors and elect the basket of securities they find to be more favorable. Consequently, the plan sponsor avoids the trading costs associated with cashing out, and the insurer avoids the costs associated with reinvesting. The middle and jumbo markets tend to transact through an independent fiduciary, who signs off on the transaction being in compliance with the applicable regulatory guidance.
That applicable regulatory guidance, which dictates how PRT transactions must occur and ensures that the plan participants become policyholders in a safe and orderly fashion as the pension plan assets and liabilities are transferred from the plan sponsor to an insurance company, can be found in Department of Labor (DOL) Interpretive Bulletin 95-1. DOL 95-1 prescribes several requirements that must be met and upon which plan fiduciaries must opine to ensure that the group annuity provided by the PRT insurer can be deemed the “safest available annuity.” 4 In short, the safest available annuity factors that should be considered require a deep-dive look into the bidding insurer’s ability to effectively run the business it is assuming as part of the PRT transaction. The factors include:
- Quality and diversification of investment portfolio.
- Size of the provider compared to the proposed PRT contract.
- Level of the insurer’s capital and surplus.
- Lines of business and other liability exposures.
- Structure of the annuity contract (e.g., use of a separate account or other guarantees).
- The availability of additional protections through state guaranty associations and the extent of those guarantees.
- Other relevant considerations, such as the insurer’s ability to administer the annuity benefits efficiently and effectively.
What this construct effectively does to the PRT market is help ensure that the pool of PRT providers remains financially strong, profitable, highly rated, reputable and very risk aware. This acts as a natural inhibitor to overly aggressive pricing in the PRT space. We should not be tempted (and, as actuaries, it is indeed our professional duty to not be tempted) to think that DOL 95-1 compliance is simply a “cover your tracks” or “check the box” exercise. Approximately one year after a high-profile sponsor’s large 2012 PRT transaction closed, there was a class-action lawsuit brought by a group of retirees who questioned the legality of the fact that the checks they used to get from the plan sponsor were now coming from a PRT insurer. The case was thrown out of court, with the primary reason being the lengths to which parties to the transaction went to ensure DOL 95-1 compliance (and the documentation of such compliance). 5
State Guaranty Association (SGA) Coverage
Focusing for a second on the sixth factor in the DOL 95-1 list of considerations provided above, in the United States, should a licensed insurer become insolvent and be liquidated by the National Organization of Life and Health Guaranty Associations (NOLHGA), after liquidation, policyholders holding policies insured by the liquidated insurer are made whole up to certain limits. These limits vary state by state (the large majority are in the $100,000–$500,000 range, with the most common being $250,000),6 and should be applied on a policy-by-policy basis to the present value (PV) of the policy’s expected future cash flows using market consistent assumptions. Smaller benefit annuities for older lives will have, of course, lower PVs relative to larger benefit annuities for younger lives. The lower the PVs are, the more likely it becomes that they would be under the state guaranty association (SGA) limits, which would help ensure that policyholders are made whole in the highly unlikely event of an insurer liquidation.
What this likely means for the PRT market is that, since there tends to be more SGA protection available for older lives with smaller annuity benefits, it is easier to gain comfort with the DOL 95-1 “safest available annuity” definition for these lives. Consequently, transactions with large groups of these lives tend to be a bit less complicated from a DOL 95-1 perspective.
We’ve seen a trend in the U.S. PRT market toward transactions in which blocks of retirees with small benefits are carved out and transferred to insurers, while the plan sponsor continues to retain the remaining plan participants. Actuarially speaking, the valuation of these carved out blocks of retirees is not complicated, which can be a good thing from one perspective; however, it doesn’t help the insurers to differentiate themselves from a pricing standpoint. It often becomes a contest of who can model mortality (as the next section of this article lays out, often in 15 days or fewer) better than everyone else. When you ask that question to a number of teams of seasoned actuaries, there’s not a lot of room for standing out in such a short time frame without uncomfortable levels of uncertainty. Predictive modeling could be a cure for that.
Predictive Modeling in the Context of PRT Transactions and How That Might Work
The illustration in Figure 2 shows a typical transaction timeline for a PRT deal in the United States in the current market. Typically, there is approximately a two-week time frame between the time the census data is delivered to the bidding insurers (around day 30) and the time that final bids are due (around day 45). Insurers generally have their mortality assumptions prepared and ready to go before receiving the census and apply those assumptions to the census when they receive it to determine pricing. There is simply not a lot of time for studies or application of different scalars based on population characteristics to meaningfully affect pricing. We usually see different tables being applied based on a handful of industry standard factors, such as age, sex, blue collar/white collar, region of residence and so on.
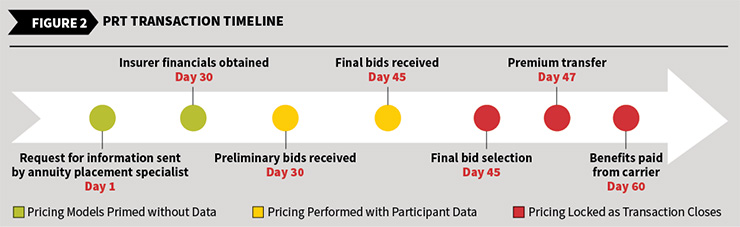
What if there was a way to gather more data on the census? Lots more. And what if an evaluation of the predictive power of that data could be accomplished overnight? In today’s world of big data and formidable predictive modeling, this can unquestionably be accomplished. The question is how it might be included as a key ingredient in PRT pricing.
Let’s say, for example, that two men lived next to each other and worked next to each other, and the first guy (“Oz”) was in PRT census No. 1 and the second guy (“Ozzie”) was in PRT census No. 2. Let’s assume, with respect to the factors PRT pricing actuaries look at to determine which mortality assumptions to apply in their PRT pricing (age, sex, etc.), Oz and Ozzie are identical.
However, when we look at the big data set, we see that Oz shops at a health food store, owns a racing bike store, is on the board of a local hiking club, has never gotten a speeding ticket, gets annual physicals and takes no medications (other than the multivitamins he buys at Whole Foods), while Ozzie runs a store that sells kegerators, eats fast food 10 times a week, has extraordinarily high cable bills, and has been on and off cholesterol medication.
That type of information has been used by predictive models in the health insurance world to inform premium pricing for many years, where the probability of contracting cancer, heart disease, diabetes and many other illnesses is taken into account by the pricing actuaries. As time goes on, that kind of information is also informing underwriting, pricing and marketing decisions in the life insurance space more and more.
Going back to our example, if census No. 1 had 10,000 people like Oz and census No. 2 had 10,000 people like Ozzie, from the perspective of a PRT insurer, census No. 2 would be much more attractive to bid on and census No. 1 might be a deal that should be avoided. We can clearly understand that logically and intuitively, but predictive modeling helps us quantify it by producing health scoring, which leads to mortality/longevity scoring. Based on that scoring, which, again, can be produced very quickly (which is needed in the context of a live PRT transaction given the tight time frame), a PRT insurer can decide whether to be a bit more aggressive with pricing or walk away from a deal. In either case, the insurer can better gauge its comfort level with the mortality assumptions it is using.
If I Were a Betting Man…
The PRT market in the United States is growing at a modest rate. Predictive modeling is permeating the life insurance space at a similarly modest rate. The eventual marriage of the two seems to be a foregone conclusion. The challenge actuaries are faced with is getting the math right. How, specifically, can we use the predictive modeling score results on a PRT census to adjust our mortality assumptions? That predicament is real, but inertia (i.e., we’ll never be able to get our arms around the math) should not be an answer for us.
As life actuaries, we should begin to understand that “the now” can be equally as powerful as “the then.” We should embrace the art of the possible and get to work on proving to ourselves that predictive modeling can help reshape the way we think about mortality and longevity risk. We should welcome the fact that the confluence of skill sets required—pension liability valuation expertise, life insurance annuity valuation expertise and statistical modeling proficiency—is uniquely in our wheelhouse. Let’s help our industry evolve.
References:
- 1. Kessler, Amy, William McCloskey, and Arnaud Bensoussan. The Pension Risk Transfer Market at $260 Billion. Prudential. http://institutionalinvestor.com/images/416/The%20Pension%20Risk%20Transfer%20Market%20at%20$260%20Billion.pdf. ↩
- 2. Deloitte CFO Survey. February 2016. ↩
- 3. “Premium Rates.” Pension Benefit Guaranty Corporation. http://www.pbgc.gov/prac/prem/premium-rates.html. ↩
- 4. https://www.dietrichassociates.com/pdf/DOL.pdf. ↩
- 5. Manganaro, John. “Verizon Retirees Take PRT Challenge to Supreme Court.” PLANSPONSOR. February 5, 2016. http://www.plansponsor.com/verizon-retirees-take-prt-challenge-to-supreme-court/. ↩
- 6. State Laws and Provisions Report. National Organization of Life and Health Insurance Guaranty Associations. July 1, 2016. https://www.nolhga.com/factsandfigures/main.cfm/location/lawdetail/docid/8. ↩